Comparison of statistical methods for ranking the importance of model and parameter uncertainties: a case study with numerical flow simulations of CO2 sequestration
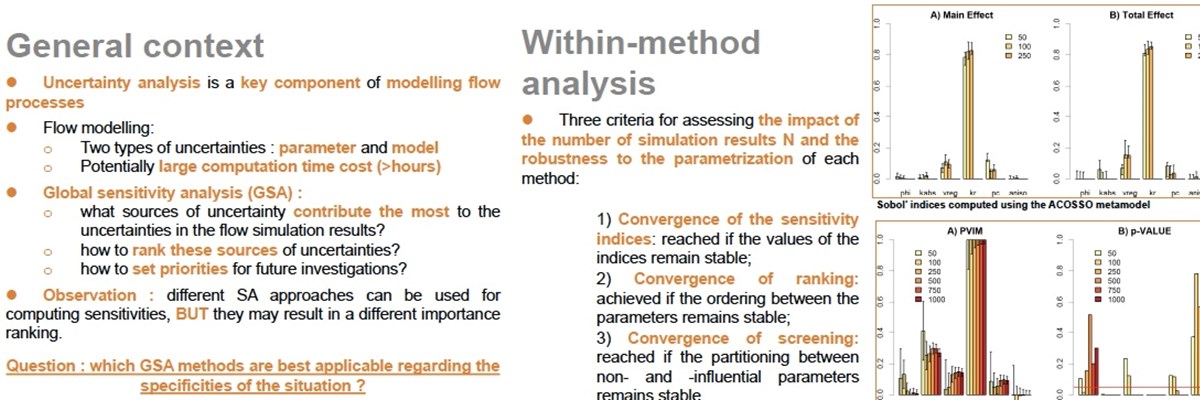
by Jean-Charles Manceau, Jérémy Rohmer and Pascal Audigane
Uncertainty analysis (sensitivity analysis [SA] and uncertainty quantification [UQ]) is a key component of modelling flow processes. Within the flow modelling workflow, several types of uncertainty sources exist; the authors focus in the present study on those most commonly encountered in practice, namely parameter and model.
(poster presented at the EGU conference 2018)
For analysing sensitivity to both parameter and model uncertainties, there is to the authors’ best knowledge often no consensus on which methods are best applicable regarding the specificities of the situation. The objective of the present study is therefore to test the feasibility of the available methods/approaches for dealing with global sensitivity analysis [GSA] with respect to parameter and model uncertainties.
Four types of approaches are tested on a dataset of 1000 numerical reservoir flow simulations taken from the study of Manceau and Rohmer (2016, DOI 10.1007/s10596-016-9588-x), which was dedicated to evaluate the fate of the CO2 injected in the lower Triassic sandstone formation in the Paris basin (France):
- DGSA: a distance-based generalized sensitivity analysis approach based on the regionalized sensitivity analysis method;
- PAWN: a density-based GSA (aka moment-indepedent);
- M-VBSA: a combination of variance-based GSA and metamodeling techniques adapted to situations using continuous and categorical variables;
- RF: a machine learning approach based on the random forest technique.
Six uncertain input parameters have been considered (porosity, permeability, permeability anisotropy, regional hydraulic gradient, relative permeability and capillary pressure), two characterized by parameter uncertainties and four characterized by model uncertainties.
The results of the SA considering in turn each method (within-method analysis) have been evaluated by investigating the impact of the number of simulations as well as the robustness to the parametrisation of each method. The comparison between methods has been done in two ways: 1) from a quantitative point of view, with the comparison of normalized sensitivity measures, and 2) from a practical point of view, with the comparison of the implementation requirements, and of the capacities of each approach.